Experian, a Big Three credit bureau offering data analytics and other services, has launched a generative AI agent called Experian Assistant that it said can cut the time it takes to develop financial models from months to days and even hours. It is integrated into Experian’s Ascend Technology Platform, which uses machine learning for data analytics in fraud detection and prevention, risk management and customer insights.
The AI Innovator recently sat down with Shri Santhanam, executive vice president and general manager of software, platforms and AI at Experian North America, to find out how Experian Assistant works, what agentic AI structure it uses – and whether they’ve solved the problem of hallucinations in gen AI assistants.
The following is an edited version of our conversation.
The AI Innovator: What is Experian Assistant?
Shri Santhanam: Companies are looking to leverage generative AI to increase the impact of their data for businesses and consumers. The Experian Assistant is a generative AI-powered assistant that helps our customers leverage the power of data. It’s like having one of our foremost experts on data and analytics available to you, 24/7.
It’s kind of like having a ChatGPT alongside you?
In a sense, yes. But when you think about generic models like ChatGPT and others, they tend to hallucinate, and give you answers which are either not precise enough or specific. What we have done is we’ve used some of that same technology, but we have created an assistant which is a deep expert on Experian’s data and gives precise and accurate answers to questions on how to reference our data and how to use this data.
It also advises data scientists on how to use the data for analytics. Now, in addition to answering questions, it also provides assistance on carrying out analytics. Our vision is, in the future, it will carry out more of these analytic tasks, operating almost as an agent on behalf of many of our customers.
What does it do exactly? Is it an assistant for your Experian Ascend platform to make it easier for people to interact with it?
Yes. There are several hundreds of thousands of rows of data, several thousands of columns, that an analyst or data scientist needs to be a deep expert on. They need to understand consumer attributes. They need to know when to use certain columns to build underwriting models, and when certain data can be regulated by the FCRA (Fair Credit Reporting Act) or not.
Well, this is time-consuming. If I’m a data scientist trying to answer questions like, ‘What do I use to build the best underwriting model? Can I use a customer attribute like their number of bankruptcies in the last year?’ These are detailed questions which are time-consuming (to answer).
The Experian Assistant makes answering these questions near instant and brings down the time to build underwriting models by 75% (according to one client). It’s faster, offers higher accuracy, and the analytical tasks can be done in a much more productive way because they reduce toil.
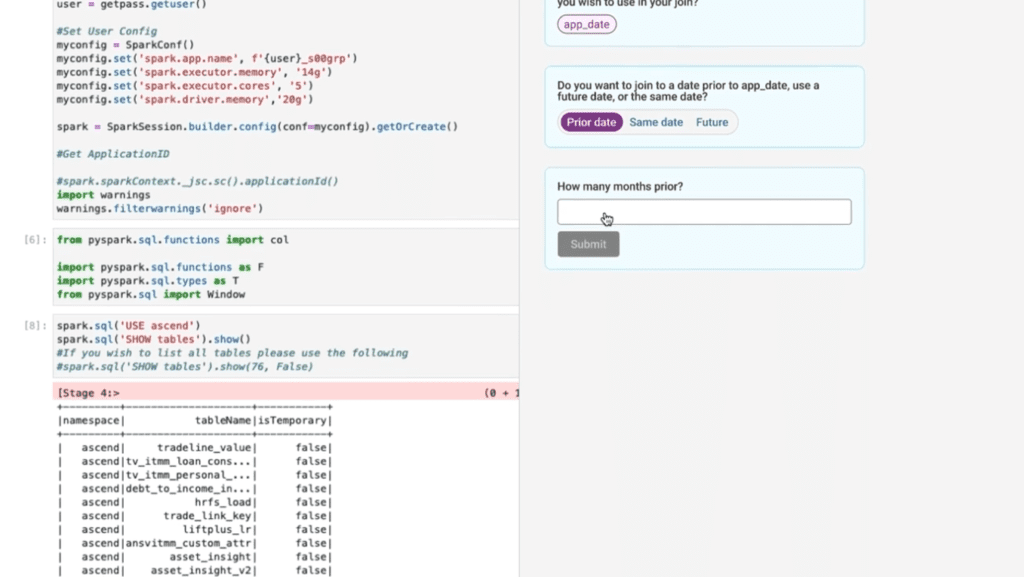
Further, it also helps democratize this capability. In the past, only deep experts in some of our data and analytical techniques could use the sandbox (to experiment with new tools), and it required a lot of training. But now with this (AI assistant), we expect a broader number of even citizen data scientists to be able to use and take advantage of some of these capabilities.
Finally, it reinforces safety, regulation, and it brings expertise to everyone’s fingertips, which ultimately leads to faster innovation for our clients and greater financial inclusion for consumers. That’s a win, win, win. It’s a win for many of our customers, for financial institutions and lenders, and it’s a win for consumers who are benefiting from greater financial inclusion.
Since you’re using AI, can you look past the traditional metrics when assessing someone’s creditworthiness?
Absolutely. There are about 28 million people in the U.S. who are ‘credit invisibles.’ When you look at traditional data and traditional scoring methods, these individuals cannot be scored. That obviously creates and exacerbates our financial inclusion problem. By expanding the universe of FCRA data that can be used, which are of course FCRA compliant, and by using more sophisticated AI/ML driven techniques, what we find is about 96% of the U.S. adult population can be scored, up from 81% – and this obviously creates greater financial inclusion. … The Experian Assistant allows our clients to understand how to use these data assets and how to use the advanced AI/ML techniques to enable the (credit scoring of ‘invisibles’).
What is the adoption rate of Experian Assistant among your clients?
There are two parts to the question you’re asking. First, on AI and machine learning and some of the more advanced techniques, (we see adoption of the technology by) the larger, more sophisticated financial institutions who have made investments in the talent, capabilities, and required regulatory investments to be able to do this in a safe and responsible way.
But we’re also seeing greater adoption around mid- to smaller financial institutions. We have products like Ascend Intelligence Services, which brings the capabilities of machine learning across the board, even to mid- and small-size financial institutions that may not necessarily have the ability to invest the way some of the largest banks in North America do.
As for the Experian Assistant, it’s early days, but we’re actually seeing very promising adoption from our early customers, and they’re starting to see it drive a higher accuracy of answers. They’re starting to see it really reduce the time it takes to build models – so very promising results so far.
How do you handle gen AI hallucinations?
Over the last several months, that’s probably been our biggest focus. When you’re providing answers in ChatGPT-like use cases where it’s more exploratory and recreational, hallucinations or imperfections in the answers matter less. But when you’re actually working with data in regulated use cases, like we are, accuracy and safety standards become much higher.
The approach we took was to define a clear set of skills, domains and areas, with a very rigorous feedback loop of how the architecture of our AI agents and solutions work. We use techniques like Retrieval-Augmented Generation (RAG), agentic frameworks and few-shot prompting to ensure … that the agents will be able to give the right answers and in the right form.
Is there an error rate that is acceptable when using AI models?
Accuracy is a really important measure for us, and we measure it in a number of ways. It isn’t as simple as quantifying the error rate, because we’re talking about very detailed, and in some cases, subjective uses. So the way we handle that is we have a panel of our experts auditing and reviewing all of the answers, and these have to meet the threshold of standards of what we believe is acceptable. Only when the (AI) agents clear that (threshold) do we allow them to answer questions.
You mentioned agentic framework. What part of Experian Assistant is agentic?
When you ask the Experian Assistant a question, what it actually does is it decides what the topic of your question is, and internally passes it over to a set of experts, which are experts in specific portions of that answer. For example, there might be an expert on answering questions for certain categories of data, there might be an expert in answering certain questions around certain types of analytics.
We’re using a framework which allows us to build this in a modular fashion over time, so we can add more agents and experts in the background, managed by a concierge, where the concierge will first look at your question, understand it, greet you, and then decide in the background which of our experts it wants to answer that question and then give you the answer.
When you say experts tapped by the Experian Assistant, do you mean it accesses a domain-specific database?
A domain-specific agent. It’s a system, basically. It could be a combination of a language model with a RAG framework with data in it. It’s an entity, if you will. … The Experian Assistant uses an agentic framework so that we can make it extensible over time to a variety of use cases.
Is that a little scary, though, to have autonomous AI agents able to do things like execute tasks for you?
The responsible use of these methods is paramount as we think about these use cases. For everything we’ve done here, that’s been a key priority and a guiding principle on how we’ve built these. We’ve designed this architecture and framework to ensure that it’s compliant with all regulation and data privacy laws – for instance, in how it handles any sensitive information.
We feel very comfortable that given our proprietary work in this area, to bring some of these innovative capabilities in a safe way is certainly a differentiator, and we’re quite excited about it. The exact point that you’re making on the caution among financial institutions is exactly the reason why we think the Experian Assistant is a powerful product and technology to be able to bring this in a safe way, in a space that’s understandably cautious.
How can you ensure that the actions of these AI agents are transparent and explainable?
When the AI agent gives an answer, we have traceability back to the sources of information it used to produce those answers. This can be checked and verified by humans. … Human in the loop verification and tier traceability are important principles for us to ensure that this AI is explainable.
Can you share some Experian Assistant use cases?
The first use cases have largely centered on better understanding our data. The second has been for modeling and reducing the modeling lifecycle. The third has been to reduce the time and toil involved in tackling tasks. Over time, we expect the set of use cases that the Experian Assistant – or the set of agents that make up the Experian Assistant – to expand over time. This will include areas like fraud and decisioning more broadly, and we’re quite excited about that vision. We see tremendous potential with this capability.
How did Experian come up with the idea of the Experian Assistant and its agentic framework? Can you describe your creative process?
One of our key principles with generative AI is we believe in unlocking grassroots innovation. We exposed many people to tools where they could start innovating in a safe way. We had some of the generative AI tools in the hands of our data scientists or developers.
This idea was born out of internal innovation. We had a set of data scientists, encouraged by some of the leadership, who brought this idea to me and the rest of our leadership team. We provided them with support from some of our technical teams (and) surrounded them with the right expertise, help and the right sponsorship and allowed it to bloom. Now, in record time, in less than a few months, we’ve gone from idea to a live product that’s in the hands of a few customers.
That’s really amazing because a lot of companies struggle with internal bureaucracies. They are resistant to change. How is Experian able to unlock innovation?
One of the first things we did was focus on AI. The conviction came from the top, right from our executive team to our CEO, and we all agreed that we needed to focus on generative AI. So we set out and set up a risk council under the sponsorship of our North American CEO, Jennifer Schulz.
The establishment of this governance framework allowed us to move quickly, but also in a responsible way. One of the challenges we observed in financial services is that there are great ideas, but given some of the concerns around risk and regulation, the ideas remain PoCs (proofs of concept) and many financial institutions are still stuck in PoC land.
For us, an important definition of success was production at scale and actually having these things in the hands of our customers. So we established this risk council, which allowed us to bring in some of these ideas in a responsible way. We have back-and-forth dialog, and mutual education among technologists, AI practitioners and other folks, which allowed us to really move at pace and go from idea to production at scale.
The second thing we’ve done is we realized that to get to production at scale, we need to build a gen AI platform. So we made an investment in building a generative AI platform, a robust technological framework which allowed us to really build some of these use cases fast, in a responsible way.
The generative AI platform is an expansion and extension of the Ascend technology platform. But in the last year and a half, we’ve brought a set of capabilities within our platform to also expand and build generative AI use cases. That was a concerted decision and an investment.
Can you compare the benefits of using Experian Assistant versus the old way of doing it?
Faster time to modeling, faster analytics, lower cost and greater productivity. Over time, we also expect that our customers will be able to innovate more and there’s also greater financial inclusion for consumers.