Key takeaways:
- Only 22% of respondents surveyed said their companies are unlocking value from generative AI adoption, according to a report by Forrester and SoftServe, a global IT consulting and services firm.
- The biggest blockers are the following: struggles with data preparation, lack of technical expertise and grappling with governance and risk management issues.
- Nearly all respondents are using a commercial foundation model (like GPT-4) and 88% have hired a cloud computing provider to host their data and train gen AI models.
The euphoria that greeted ChatGPT in late 2022 came with high hopes of operational transformation throughout the enterprise. But a report released today revealed the real picture: Organizations are struggling to adopt generative AI even though most believe it will give them a competitive advantage.
Just 22% of respondents surveyed said their organizations are unlocking value from generative AI adoption, according to a report from Forrester commissioned by SoftServe, a global IT consulting and digital services provider.
It’s not from lack of interest. More than 80% of respondents believe gen AI will rise in importance in the next 12 months. Over half see gen AI as a strategic business asset for transformation.
It’s also not from lack of trying. Nearly all (95%) are using a commercial foundation model (such as GPT-4) and 88% have hired a cloud computing vendor to host their data and train gen AI models. Also, 92% said they either fine-tune pre-trained models, or train their gen AI models, with their own data.
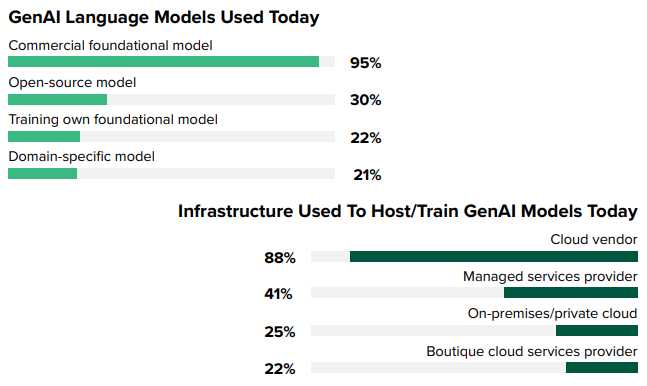
So what’s the problem? Nearly 90% struggle to prepare enterprise data for AI models and 79% said they were “concerned or very concerned” they do not have enough technical expertise to meet their gen AI goals. Three-quarters are grappling with governance, risk management, privacy and security issues.
“Despite a swift start to the Gen AI race, many initiatives get stuck in the piloting stages as more organizations realize their data infrastructure isn’t ready to adequately deploy Gen AI technologies beyond the proof-of-concept,” said Alex Chubay, SoftServe’s CTO, in a statement.
“Gaps in skills and knowledge of emerging Gen AI technologies, technical feasibility, and data readiness hinder companies from moving beyond tactical wins in pilot mode to full-scale deployments enabling novel business capabilities and experiences,” he added.
Forrester surveyed 777 decision-makers globally that make technology purchases and who are involved in their companies’ gen AI efforts.
What they’re doing now
Companies are deploying use cases, but struggle to identify which ones can deliver the “most immediate, impactful value” across the organization. But their challenges aren’t stopping them from trying: On average, respondents have fully implemented three use cases, are working on expanding to two more and plan to pilot one in the short term.
The most common use cases are intelligent employee support, enhanced customer engagement, assisted developer support, automated insights discovery, and intelligent enterprise search.
However, many cannot connect foundation models to the full range of enterprise data. Nearly 90% said their companies need help consolidating and streamlining enterprise data for use in gen AI models.
On average, respondents were able to leverage just three types of data – operational, customer and employee data. A quarter of respondents can leverage four or more types of data while just 3% can tap the full range of data (operational, customer, employee, source code, public and partner data.)
The retail industry was “much more likely” to get value from gen AI (value reaper), while financial services firms were “much less likely” (value seeker), the report said. Retailers also were more likely to have trained their own gen AI models on their own data than financial services firms. Respondents in the health care, life sciences, oil and gas, manufacturing, and enterprise technology industries, as well as independent software vendors, were evenly split between value reapers and seekers.
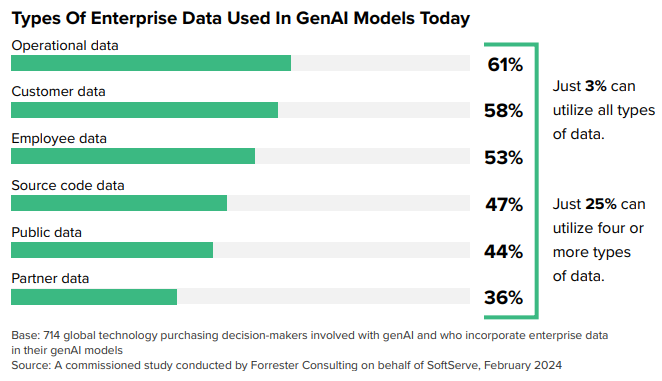
Governance gap
A full 90% of respondents believe a governance plan is important to ensure gen AI is deployed responsibly and complies with regulations while leading to desired business outcomes. However, just 24% have instituted governance plans. This gap exposes organizations to risk.
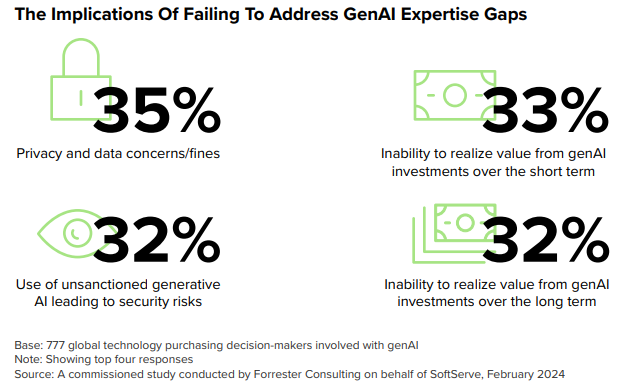
What success looks like
Companies that successfully leverage gen AI to produce value have the following characteristics:
- They were more likely to use a commercial foundational model and a cloud vendor to host their data.
- They have more advanced data capabilities and skills to full train models on their organizations’ data. They are better at preparing, managing and leveraging four or more types of data for gen AI models.
- They are more likely to have a governance policy, understanding it contributes to gen AI’s success.
- They realize that deeper technical expertise is key to data integration, model optimization, use case development and further app development. Thus, they focus on improving their technical skills.
- They look for partners that can help accelerate deployment, have advanced technical capabilities and possess a deep understanding of their market.
For companies seeking to improve deployment, the report recommends the following actions:
- Update ROI and impact assessments periodically as your organization matures. It will let you more accurately assess value and understand your company’s changing needs.
- Make data preparation a foundation of your strategy. Preparing data for gen AI applications include generative questions and answers for fine-tuning; ingesting and indexing documents for RAG (retrieval-augmented generation); or unsupervised fine-tuning. Understand what data preparation you need to do when choosing gen AI use cases that will add value.
- Track progress in organizational readiness and skills. Employ continuous learning in the company.
- Find external partners to help you bridge gaps in expertise.